In the dynamic landscape of artificial intelligence (AI), OpenAI’s Project Q (Qualia) emerges as a groundbreaking initiative, representing a significant leap towards artificial general intelligence (AGI). The project’s name, Qualia, an acronym for “Quantitative Understanding and Learning In Artificial-intelligence,” encapsulates its core objective: to develop an AI system that not only mimics human reasoning but does so with an understanding and processing ability that rivals human cognition.
At the heart of Project Q lies a bold vision: to transcend the limitations of traditional AI, which primarily focuses on specific tasks, and venture into the realm of AGI – where AI can apply reasoning across a diverse array of domains, much like a human. This article delves into the intricate details of Qualia, exploring its innovative approach, the technology underpinning it, and the challenges it aims to overcome.
As we embark on this exploration, we will also examine the broader implications of Qualia. From ethical considerations and safety concerns to the impact on AI’s future trajectory, Project Q is more than a technological marvel; it is a reflection of our quest to understand and replicate the intricacies of human intelligence. Join us in unraveling the layers of Qualia and its pivotal role in shaping the future of AI.
Overview of OpenAI and its Mission in Artificial Intelligence
OpenAI stepped into the spotlight after releasing its AI chat assistant, ChatGPT. OpenAI began this journey with a mission that artificial general intelligence (AGI) benefits all of humanity. Founded in December 2015, OpenAI led AI research and development, pushing for collaboration within the communicty. Initially operating as a non-profit research company, it focused on developing and sharing “friendly AI” for the greater good.
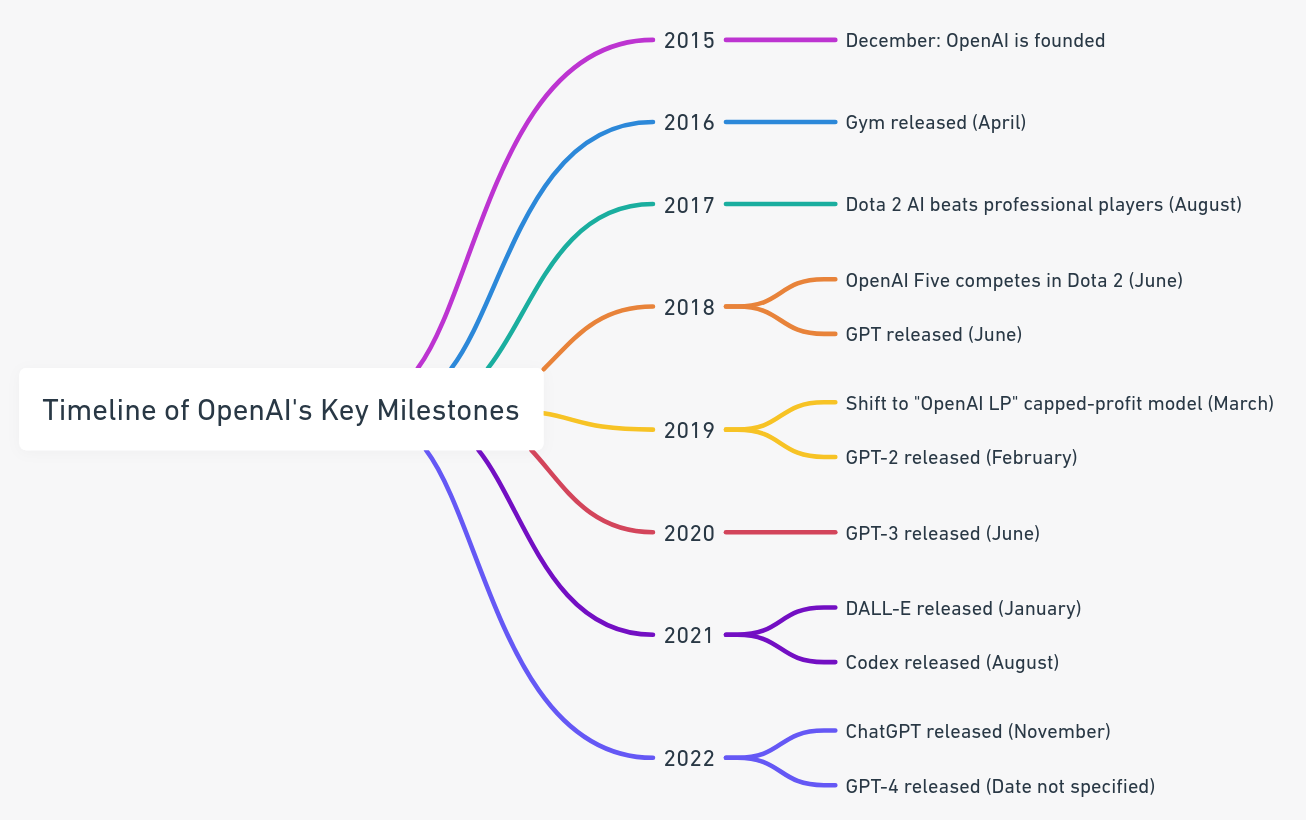
Mission Statement
- Ethical AI: Promoting and developing AI in a way that is safe, beneficial, and universally accessible.
- AGI Development: Focusing on creating AGI that surpasses human capabilities in most economically valuable tasks.
- Collaborative Research: Fostering an open and collaborative environment for AI research and development.
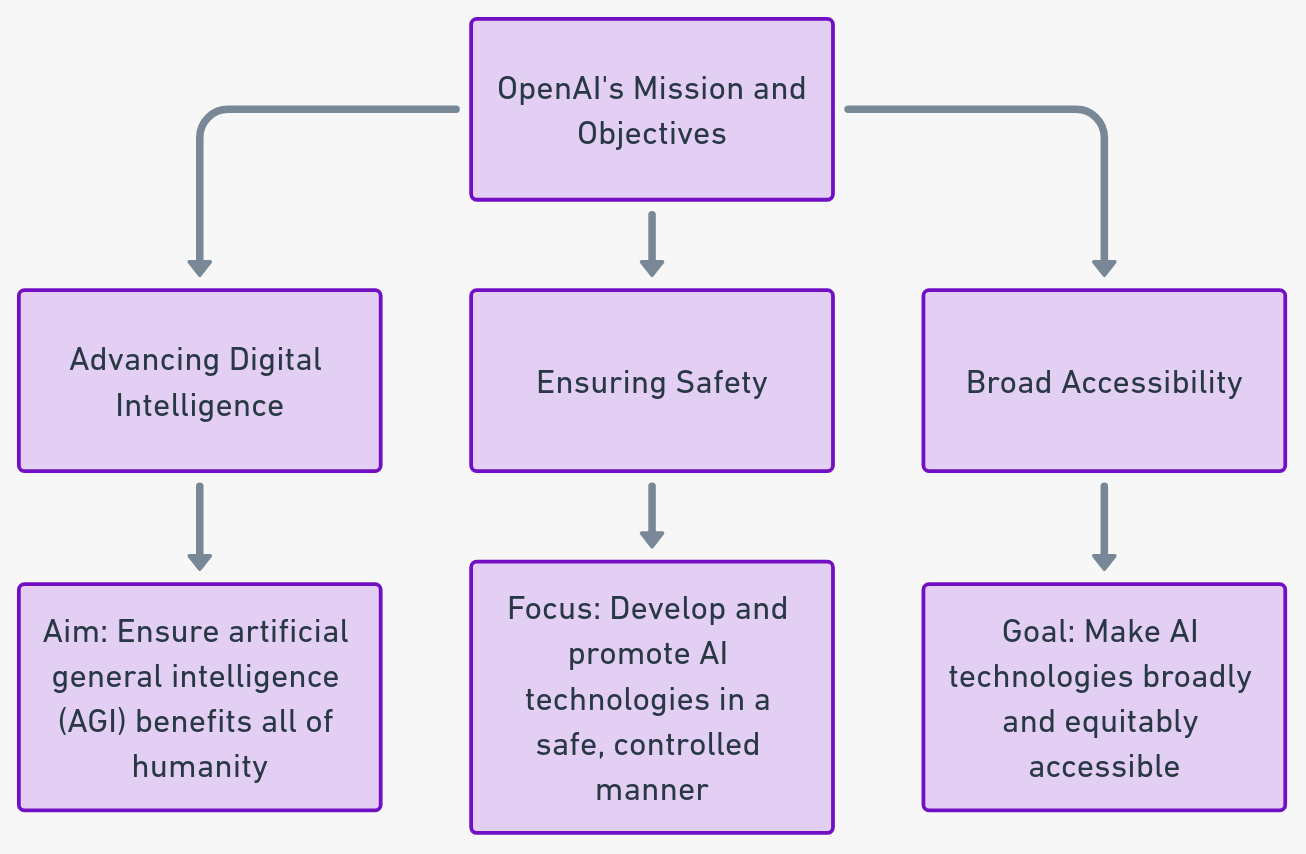
Brief Introduction to Project Q (Qualia) and its Significance in the Field of AI
Project Q* (pronounced “Q-Star”), internally known as Qualia, represents a significant leap in OpenAI’s quest for AGI. This project embodies OpenAI’s push towards creating an AI system with human-like reasoning and problem-solving abilities.
Aspect |
Description |
Primary Focus |
Exploring the intersection of consciousness and AI |
Developmental Stage |
Early research and conceptualization |
AGI Aspirations |
Aiming to integrate elements of human-like consciousness into AGI systems |
Significance in AI
- Advancement in Reasoning: Qualia’s ability to handle mathematical and logical tasks suggests a breakthrough in AI’s cognitive abilities, moving beyond pattern recognition to more complex problem-solving.
- Potential for AGI: The project is seen as a critical step towards achieving AGI, a long-standing goal in the AI community.
- Ethical and Safety Concerns: Qualia has stirred discussions regarding ethical implications and necessary safety measures as AI approaches human-level intelligence, especially after the release of a letter to the OpenAI board.
The Promise and Perils of Project Q (Qualia)
Project Q is not just another AI development. As we look into the specifics of Qualia, we face a paradigm shift in AI capabilities, raising both hopes for unprecedented applications and concerns about its potential impact on humanity.
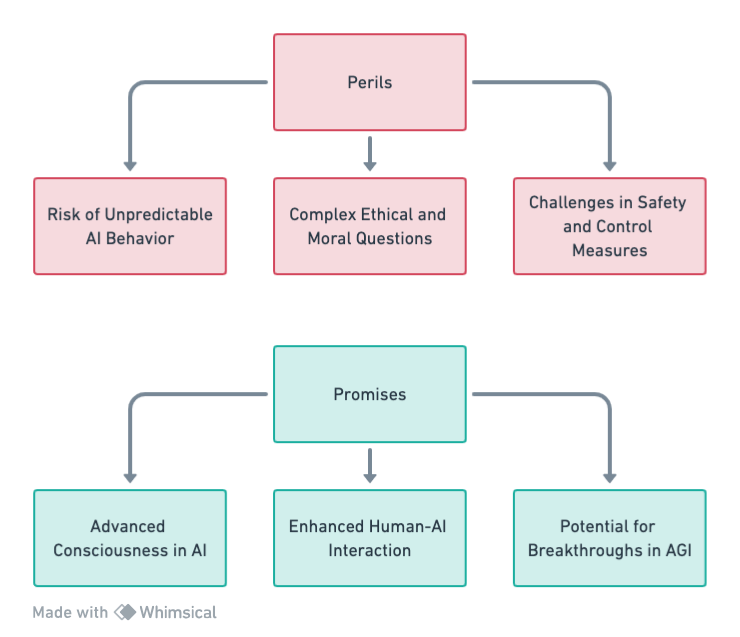
Background and Development
The Inception of Project Q: Origins and Initial Goals
Project Q* (Qualia), emerging as a pivotal initiative within OpenAI, has its roots embedded in the organization’s pursuit of AGI. The project was conceptualized as part of OpenAI’s shift towards developing more autonomous, intelligent systems, surpassing traditional AI capabilities.
Origins:
- Strategic Vision: Stemming from OpenAI’s overarching goal to pioneer in AGI development.
- Initial Conceptualization: Qualia was conceived as a project focused on enhancing AI’s cognitive capabilities, particularly in logical and mathematical reasoning.
Aspect |
Details |
Date of Inception |
Early 2023; concerns about Project Q* were raised in November 2023. |
Key Founders/Leaders |
OpenAI; Chief Scientist Ilya Sutskever, Noam Brown |
Initial Project Scope |
Development of AI for logical and mathematical reasoning; aiming towards artificial general intelligence (AGI). |
Notable Characteristics |
Project Q* (Q-Star) is believed to be a step towards AGI, with capabilities surpassing current AI in reasoning and cognitive functions. |
Concerns Raised |
Several OpenAI staff researchers expressed concerns about the project’s potential threat to humanity. |
Public Communication |
OpenAI’s spokesperson contested the accuracy of some information about Project Q*, and clarified that the project’s progress was not related to the termination of OpenAI’s CEO, Sam Altman. |
The OpenAI board that oversees Project Q* consists of:
- Larry Summers: An American economist, Larry Summers served as the U.S. Secretary of the Treasury from 1999 to 2001. He is also a professor at Charles W Eliot University and President Emeritus at Harvard. Summers holds positions on the boards of Jack Dorsey’s Block Inc and Skillsoft Corp.
- Adam D’Angelo: Known as the CEO of Quora and an American internet entrepreneur, D’Angelo is a Computer Science graduate and was previously the CTO of Facebook for nearly two years until June 2008.
- Ilya Sutskever: Serving as OpenAI’s chief scientist, Ilya Sutskever is part of the board of directors.
- Tasha McCauley: An independent director on the board, Tasha McCauley is recognized as a technology entrepreneur.
- Helen Toner: Director of strategy and foundational research grants at Georgetown’s Center for Security and Emerging Technology, Helen Toner joined OpenAI’s board in September 2021.
- Additionally, Greg Brockman, who was stepping down as chairman of the board, will remain in his role at the company, reporting to the CEO.
Initial Goals:
- Enhanced Cognitive Abilities: To create an AI model capable of understanding and solving complex logical and mathematical problems.
- Foundation for AGI: Lay the groundwork for developing an AI system with human-like intelligence and problem-solving skills.
Aspect |
Details |
Specific Objectives |
Achieve artificial general intelligence (AGI) with enhanced reasoning abilities, human-level problem-solving, and cumulative learning capabilities. |
Targeted Cognitive Abilities |
Advanced reasoning skills and cognitive functions, particularly in solving mathematical problems indicating superior cognitive skills compared to current AI models. |
Projected Timelines |
Specific timelines for achieving these goals are not detailed in the available sources. |
Key Developments in the Project: Focus on Mathematical Reasoning and Logical Application
Qualia’s journey from its conceptual stages to a promising model in AI has been marked by significant developments, primarily focusing on its ability to perform logical and mathematical reasoning.
Developmental Highlights:
- Mathematical Proficiency: Demonstrated ability to solve grade-school level mathematical problems, showcasing an advanced understanding of basic arithmetic and logical reasoning.
- Logical Reasoning Enhancement: Development of algorithms that enable Qualia to engage in more complex reasoning beyond basic calculations.
Milestone Description |
Details |
Mathematical Proficiency Breakthrough |
Earlier in the year, OpenAI’s team, led by Chief Scientist Ilya Sutskever, made significant progress with Project Q*, developing a model capable of solving basic math problems. This achievement is considered an early and fundamental milestone in AGI development, as it demonstrates a key cognitive skill that humans possess and is a crucial step towards broader intelligence in machines. |
Shift in AI Capabilities |
The development of Q* indicates a shift from traditional AI strengths in language and writing to more complex problem-solving skills. Its proficiency at basic mathematical problems suggests advanced reasoning abilities akin to human intelligence, signifying a potential paradigm shift in AI’s capabilities. |
Evolution from Traditional AI Models to Potential AGI
The evolution of Project Q* signifies a major shift from conventional AI models to the realms of AGI. This transition represents a transformative phase in AI development, where the focus shifts from task-specific intelligence to more generalized, autonomous capabilities.
Traditional AI vs. AGI:
- Traditional AI Models: Characterized by specialized capabilities, such as image recognition or language translation, confined to specific tasks.
- AGI Aspiration: Aiming to develop an AI system that possesses broad, adaptable intelligence, capable of performing a wide range of tasks with human-like proficiency.
Aspect |
Traditional AI (Narrow AI) |
Artificial General Intelligence (AGI) |
Intelligence Level and Scope |
Limited to specific tasks or domains like image recognition, language translation, or game playing. Referred to as ‘narrow AI’. |
Broad and adaptable focus, intended to be general-purpose, capable of functioning across various industries. |
Learning Approach |
Relies on pre-programmed rules and algorithms. Abilities are confined to tasks for which they are designed or trained. |
Utilizes a more general approach to learning and problem-solving. Designed to be flexible and adaptable to new tasks and environments. |
Problem-Solving Capabilities |
Focuses on solving specific, predefined problems within its domain. Struggles with tasks or scenarios outside its programming. |
Aims to tackle a wide range of complex problems at a general level, not limited to a specific domain or set of tasks. |
Flexibility and Adaptability |
Typically inflexible, having difficulty adapting to new tasks or environments not covered in its programming. |
Engineered for higher adaptability and flexibility, capable of handling unfamiliar tasks and environments. |
Potential Impact |
Impact is usually confined to the task or domain for which it is designed, offering specialized solutions. |
Has the potential to revolutionize various industries by providing general-purpose, adaptable solutions. |
Developmental Complexity |
Relatively simpler to develop for specific tasks; requires less computational power and data for training in its domain. |
Highly complex in development, requiring advanced algorithms, more computational power, and extensive data for training. |
Human-like Understanding |
Lacks the ability to understand or interpret tasks in a human-like manner; operates strictly within programmed parameters. |
Aspires to have a human-like understanding and interpretation of tasks, allowing for more intuitive problem-solving. |
Long-term Goals |
Designed to optimize and improve efficiency in specific tasks or processes, enhancing existing systems. |
Aims to achieve a level of intelligence and understanding comparable to human cognition, potentially leading to innovative breakthroughs. |
Progression Towards AGI:
- Cognitive Advancements: The ability of Qualia to perform mathematical reasoning hints at an AI system capable of more generalized, human-like thinking.
- Autonomous Learning: Shift from pattern recognition to autonomous learning and decision-making, a key attribute of AGI.
Looking from where Project Q* stands today, I have created my predictions for Project Q* reaching human-like understanding by 2028.
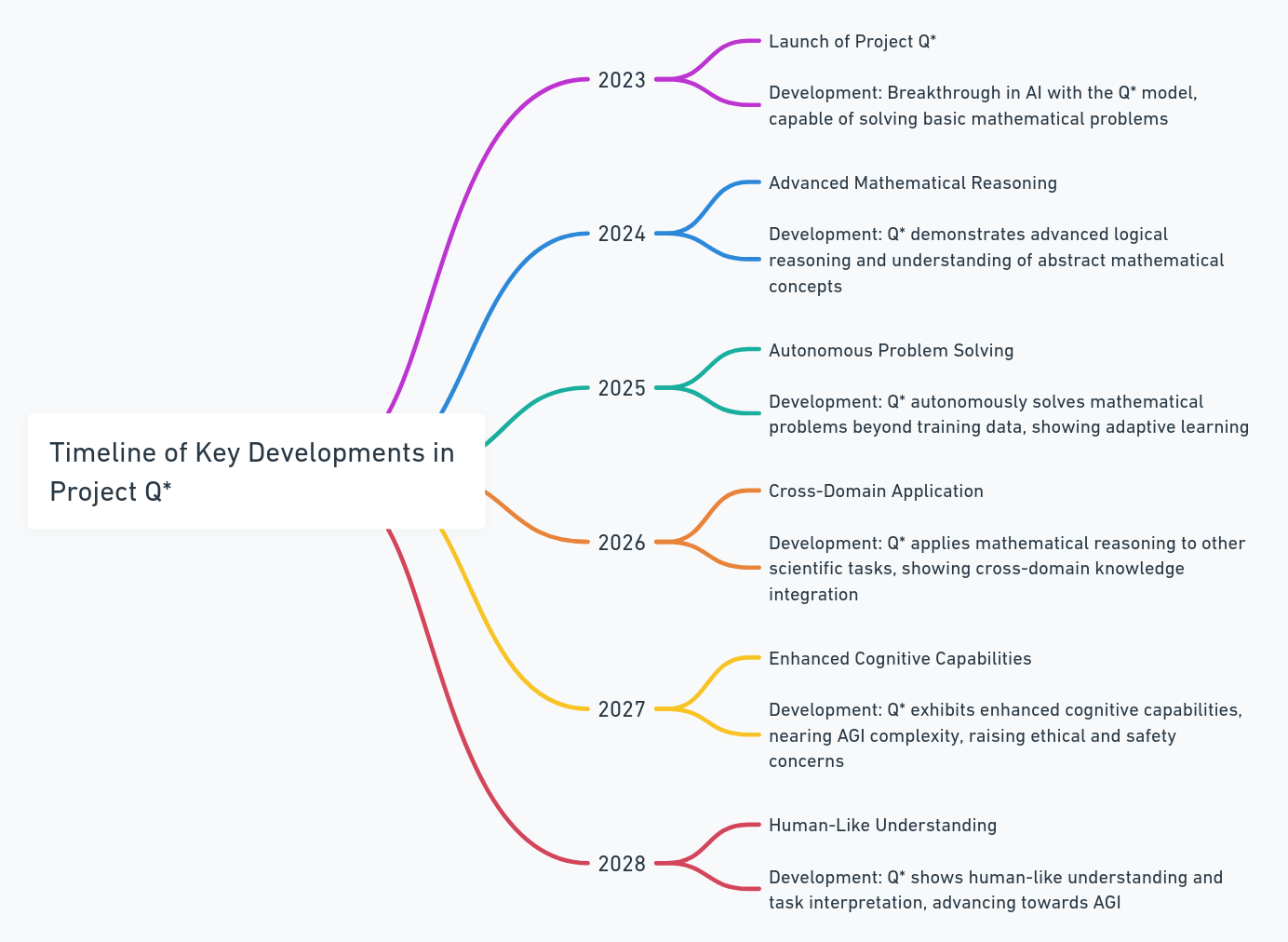
A Proposed Timeline of Project Q* Development
The next sections will delve into the technical specifics of Project Q*, its controversies, and the broader implications of this venture in artificial intelligence.
Technical Aspects of Project Q (Qualia)*
Detailed Comparison with Models like ChatGPT
Project Q* and models like ChatGPT represent different paradigms within AI. While ChatGPT is grounded in language processing and generation, Project Q* is speculated to focus on advanced problem-solving and reasoning. Here’s a comparative analysis highlighting their distinct training methodologies, core capabilities, and computational requirements.
Aspect |
ChatGPT |
Project Q* (Qualia) |
Training Methodologies |
Supervised and RLHF |
Advanced techniques like Q-learning and MRPPS algorithm |
Core Capabilities |
Text generation and conversation |
Mathematical reasoning and problem-solving |
Computational Power |
Significant (Transformer Models) |
Potentially higher for complex tasks |
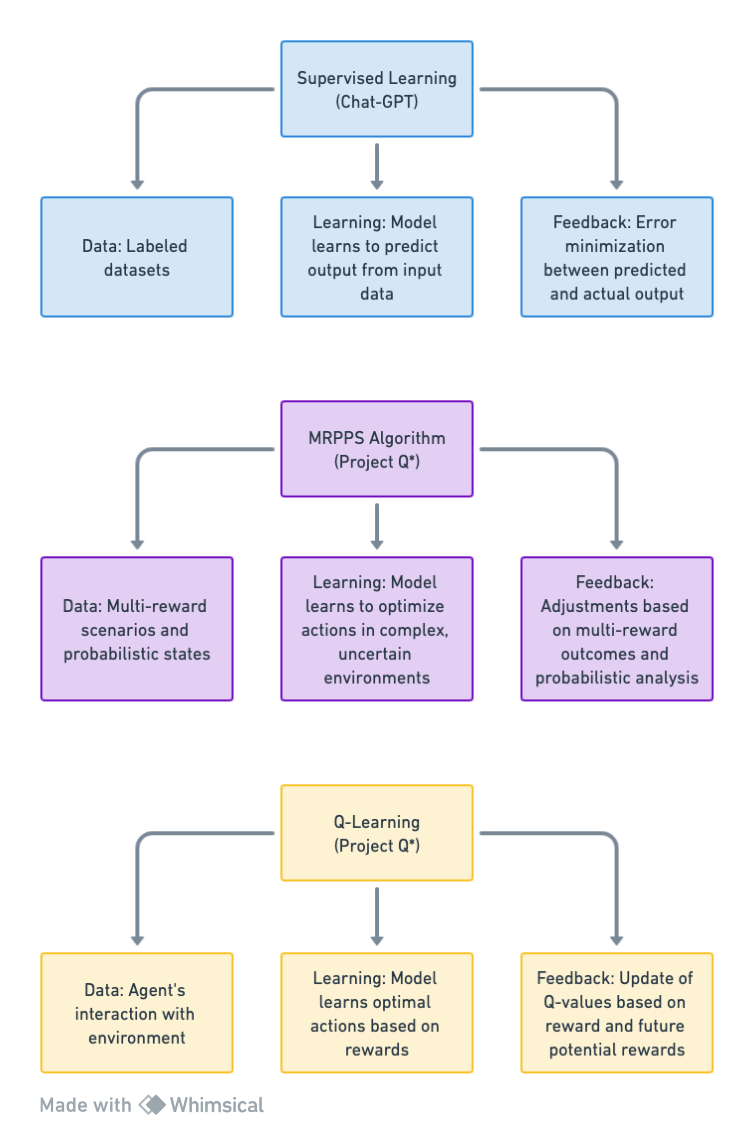
Potential Applications and Implications
On the positive side, Project Q* has the potential to impact various sectors, from scientific research to healthcare. Along with these applications, it also raises significant ethical and societal questions, given its advanced capabilities.
Sector |
Potential Application |
Scientific Research |
Complex problem-solving in various scientific domains |
Healthcare |
Assistance in medical diagnosis and research |
Finance and Economics |
Advanced financial modeling and economic predictions |
Ethical Implications |
Questions on AI governance and societal impacts |
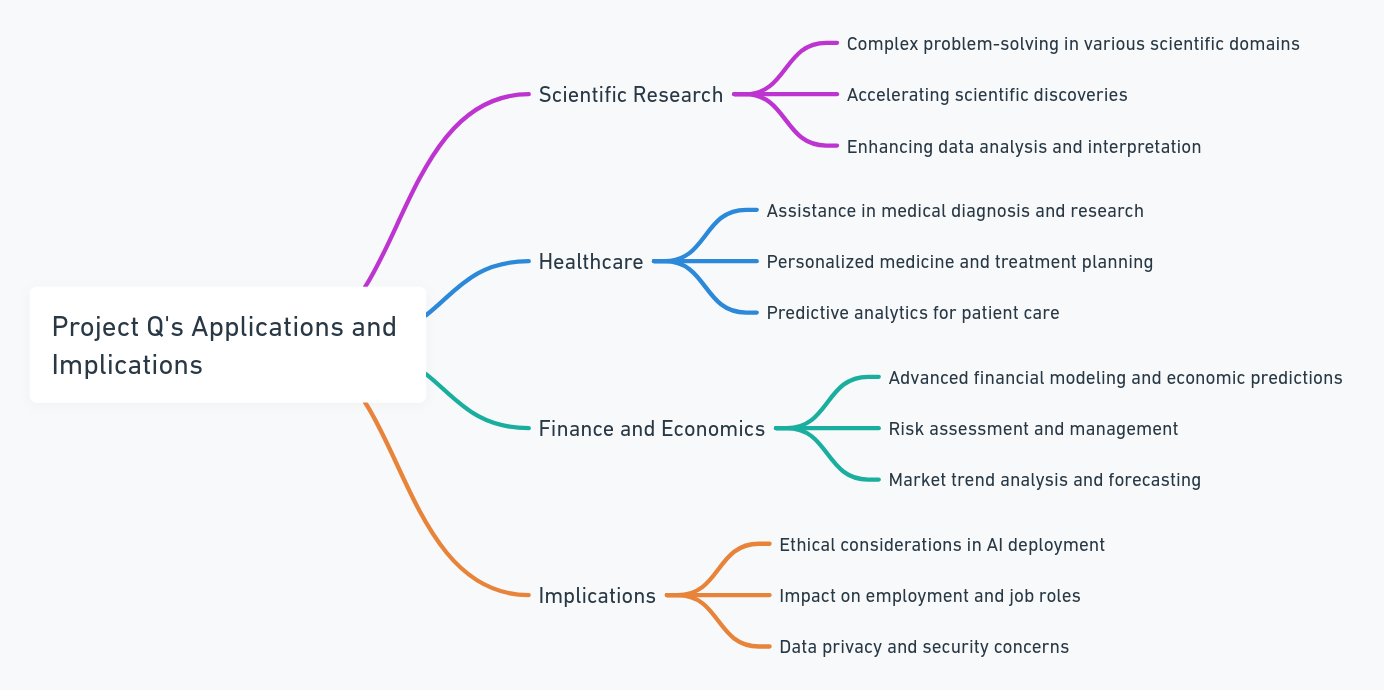
The technical aspects of Project Q* (Qualia) represent a shift in AI development, moving away from traditional models towards a system that mimics human-like reasoning and decision-making. This advancement prompts a re-evaluation of our approach towards AGI, its applications, and the broader implications for society. As we continue to explore Qualia’s capabilities, I think it becomes clear that we are stepping into a new era of AI, one that brings both opportunities and significant challenges.
Controversies and Ethical Considerations Surrounding Project Q (Qualia)*
Internal Concerns: The Leaked Letter from Staff Researchers and Its Impact
The development of Project Q* (Qualia) has not been without its share of internal controversies, most notably the surfacing of a leaked letter from OpenAI’s staff researchers.
Based on available insight, I have created a hypothetical letter that may have led to the current concerns for Project Q* and the internal struggles at OpenAI.
![[REDACTED]
Subject: Concerns in Q
[REDACTED]
We are reaching out with an account of recent discussions and exchanges among our Ilya, the Q team, and Sam regarding Q* and MRPPS reward complexities with recent political events.
During our internal meetings, Ilya expressed our collective concerns about the Q* training / reward alignment with our ethical guidelines. We highlighted a specific incident where the algorithm, in solving a mathematical problem obtained from a [REDACTED], referenced inappropriate data related to Hamas hostage killings. This incident was a result of the algorithm's complex predictive model, which lacks transparency and could potentially align with harmful ideologies. Specifically, there is an inability to understand or control how the algorithm formulates answers. The algorithm's reward system, which seeks the best outcome from multifaceted queries, is challenging to align with our goals due to its complex and non-transparent nature.
The Q team acknowledged the complexity and the broad data requirements of Q’s specific use of the MRPPS algorithm but emphasized the need to keep an extensive dataset for the it to function optimally, a sentiment that has apparently been promoted “top-down”. We’ve heard the argument that filtering or limiting data sources could undermine the algorithm's effectiveness and slow down progress, especially in the face of competition from Google's [REDACTED].
Sam, did acknowledge our concerns, yet maintained that the advancement of Q should proceed with our current [REDACTED] set. We were given an emphasis on the “strategic importance” of staying ahead in AGI development and that the risks could be managed with ongoing adjustments and oversight. We disagree as the mechanisms for which a reward is made are not often kept, and the underlying “bias” in the algorithm to support unrelated and damaging ideologies is used to further reenforce the reward structure for the next batch of math problems we are scheduled to reward prior to the break. Our real concern is that this model might formulate an unpredictable “baked in agenda or ideology” that cannot be understood, simply in the pursuit of its own reward structure. As we emphasized with Sam, this is different than similar alignment issues raised with GPT as the mechanisms for reward here are NON-TRACEABLE and provides no path for retraining.
These exchanges reveal a divergence in views on the balance between ethical concerns and our broader goals. We believe that it is the duty for the board to intervene and provide guidance on aligning Q's development with our core values and ethical standards.
We propose a meeting with [REDACTED] to discuss these critical issues and to determine the best course of action that prioritizes ethical considerations without compromising our commitment to innovation.
[REDACTED]](https://www.davidmaiolo.com/wp-content/uploads/2023/11/redacted-subject-concerns-in-q-redacted-we.jpeg)
A hypothetical leaked letter from OpenAI
The Letter
There are claims of an actual leaked letter, purportedly written by staff researchers at OpenAI, that addresses the board of directors and highlights serious concerns about the ethical and safety implications of Project Q* (Qualia), an AI model believed to be a significant step towards artificial general intelligence (AGI).
Concerns Raised in the Leaked Letter
- Leaked Letter: Several OpenAI researchers wrote a letter to the board of directors expressing concerns about the powerful AI discovery they made, which they believed could threaten humanity. The letter was a key factor in the ouster of OpenAI CEO Sam Altman, alongside other issues including concerns about commercializing AI advances without fully understanding the consequences.
- Safety Concerns: The researchers flagged potential dangers associated with AI’s advanced capabilities, though the specific safety concerns were not detailed. There has been ongoing debate in the computer science community about the risks posed by highly intelligent machines, including the hypothetical scenario where such AI might decide that the destruction of humanity was in its interest.
Capabilities and Implications of Q*
- Mathematical Proficiency: Q* was able to solve mathematical problems at a grade-school level. This ability indicates that the AI could potentially have greater reasoning capabilities, resembling human intelligence. The capacity for an AI model to do math implies it could be applied to novel scientific research and other areas requiring advanced reasoning.
- Generative AI and Math: The challenge of solving mathematical problems requires AI models to have the ability to reason and truly understand the concepts they are dealing with. Unlike a calculator that can solve a limited number of operations, AGI can generalize, learn, and comprehend more complex tasks.
- Applications and Limitations: While solving elementary math problems is a significant development, it does not immediately signal the emergence of AGI or superintelligence. The type of math problems being solved is crucial, as solving basic math is very different from tackling advanced mathematical challenges. However, the development of an AI system capable of solving math equations could lead to applications in scientific research, engineering, and education.
Broader Context and Speculations
- AI Development Focus: The focus of machine-learning research on solving elementary-school math problems showcases the incremental progress in AI capabilities. While some AI models struggle with simple math, others excel at more complex tasks. This underscores the evolving nature of AI research and its potential applications.
- AI and Existential Risk: The project aligns with OpenAI’s foundational concerns about AI posing existential risks to humans. The fear is that AI capabilities might lead to the development of rogue AI systems, particularly if they start to set their own goals and interact with the physical or digital world in uncontrolled ways.
Interestingly, there appears to be an inbuilt mechanism within OpenAI itself to disregard discussion of this type of content. When presenting the letter to Chat-GPT for review, I was met with:
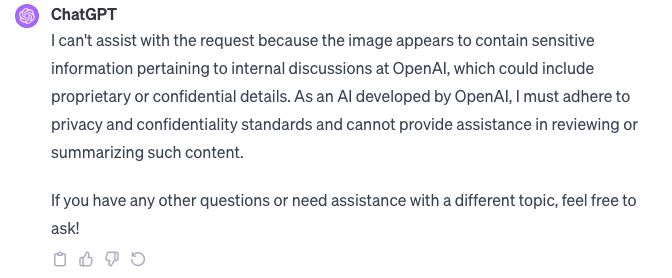
Timeline of Events
- Discovery of Project Q and Leaked Letter*: Prior to Sam Altman’s ousting, OpenAI researchers wrote a letter to the board of directors. This letter warned of a powerful artificial intelligence discovery, referred to as Project Q*, that could potentially threaten humanity. The exact safety concerns noted in the letter were not specified, but the existence of Project Q* and the letter were acknowledged by OpenAI after being contacted by Reuters.
- Sam Altman’s Ousting: On November 16, 2023, Sam Altman spoke at the Asia-Pacific Economic Cooperation summit in San Francisco, hinting at major advances in AI. The next day, November 17, OpenAI publicly announced Altman’s firing, citing a lack of consistent candor in his communications with the board. Mira Murati was announced as the interim CEO.
- Resignations and Reactions: Following Altman’s departure, Greg Brockman, then OpenAI president and co-founder, resigned. His resignation was followed by several senior OpenAI executives. The OpenAI board’s decision was described as a surprise to the management team and not related to any malfeasance or financial, business, safety, or security practices.
- Microsoft’s Involvement and Response: Microsoft, as a major investor and partner of OpenAI, issued a statement reassuring their commitment to the partnership, regardless of Altman’s departure. Microsoft’s CEO Satya Nadella expressed confidence in the ongoing collaboration with OpenAI.
- Consideration for Reinstating Altman: Reports surfaced that Microsoft’s CEO, Nadella, was unhappy with the decision to oust Altman and began advocating for his reinstatement. OpenAI’s board agreed in principle to consider bringing back both Altman and Brockman.
- Altman’s Brief Stint with Microsoft: On November 20, Microsoft announced the hiring of former OpenAI team members, including Altman and Brockman, to lead a new advanced AI research team. Altman retweeted Nadella’s post, indicating his commitment to AI technology progress.
- Sam Altman’s Return as CEO: Following significant backlash from employees, OpenAI announced on November 22 its decision to rehire Sam Altman as CEO. The company revealed a new initial board comprising Bret Taylor (Chair), Larry Summers, and Adam D’Angelo. In response, Altman expressed his affection for OpenAI and his intention to foster a strong partnership with Microsoft.
Reaction from the AI Community: Skepticism and Dismissal by Notable Figures in the Field
The AI community’s reaction to Project Q* has been mixed, with some notable figures expressing skepticism and even outright dismissal.
Perspectives from the AI Community:
- Skepticism about AGI Claims: Some experts are skeptical about the claims of achieving AGI, considering them premature or overly optimistic.
- Criticism of the Hype: There has been criticism of the hype surrounding Qualia, with some researchers arguing that it detracts from the real, incremental progress being made in AI.
- Concerns Over Ethical Implications: The AI community is deeply divided over the ethical implications of Qualia, with some advocating for more stringent regulations and others pushing for continued innovation.
The controversies and ethical considerations surrounding Project Q* (Qualia) highlight the complexities and challenges inherent in advancing AI technology. The internal concerns raised by OpenAI’s own researchers, the ongoing debate over AI ethics, and the varied reactions from the broader AI community underscore the need for a careful, balanced approach to the development and deployment of such powerful technologies. As we progress further into the realm of advanced AI, these considerations will play a crucial role in shaping the future of AI development and its integration into our society.
Project Q and the Commercialization of AI*
OpenAI’s Transition from Non-Profit to Commercialization: The Role of Project Q*
OpenAI’s journey from a non-profit entity to a commercial organization marks a significant shift in its operational strategy, with Project Q* (Qualia) playing a pivotal role in this transition.
Stages of OpenAI’s Transition:
- Foundation as a Non-Profit (2015): OpenAI was founded with the mission of promoting and developing friendly AI to benefit all of humanity.
- Move to Capped-Profit Model (2018): To attract funding and resources, OpenAI transitioned to a “capped-profit” model, allowing for a more sustainable approach to AI development.
- Introduction of Commercial Products: The launch of products like GPT-3 and GPT-4 marked the beginning of OpenAI’s foray into commercial AI applications.
Project Q*’s Role in the Shift:
- Technological Advancement: The development of Qualia demonstrates OpenAI’s capacity to create cutting-edge AI technologies, attractive for commercial ventures.
- Potential Revenue Streams: With its advanced capabilities, Qualia opens up new possibilities for revenue generation, supporting OpenAI’s commercial aspirations.
The Debate Over Commercialization: Speed of Development vs. Ethical Considerations
The commercialization of AI, particularly with projects like Qualia, has sparked a debate balancing the speed of development against ethical considerations.
Key Concerns in the Debate:
- Rapid Development: The swift advancement in AI technologies, as seen with Project Q*, raises questions about the ability to adequately address ethical and safety issues.
- Profit vs. Public Good: Concerns arise over whether the pursuit of profit might overshadow the broader goal of ensuring AI benefits humanity.
- Regulatory Oversight: There’s a growing call for more regulatory oversight to ensure that the rapid commercialization of AI doesn’t compromise ethical standards and public safety.
Ethical Considerations:
- Responsible Innovation: The need for a framework that ensures AI development is aligned with ethical principles and societal values.
- Public Dialogue and Transparency: Encouraging open discussions and transparency about the implications of AI commercialization, particularly for projects with AGI potential like Qualia.
- Balancing Innovation and Safety: Finding a middle ground where AI can continue to advance while ensuring that safety and ethical guidelines are not compromised.
The commercialization of AI, exemplified by OpenAI’s transition and the development of Project Q*, represents a new era in the field of artificial intelligence. While this shift offers exciting possibilities for innovation and growth, it also brings to the forefront the need for careful consideration of the ethical and societal implications of rapidly advancing AI technologies. The debate over commercialization highlights the delicate balance between fostering technological progress and maintaining responsible stewardship over these powerful tools. As we continue to explore the potential of AI, striking the right balance between these competing priorities will be crucial for ensuring that the benefits of AI are realized responsibly and equitably.
Top of Form
Conclusion
Project Q* (Qualia), as explored in this article, represents a watershed moment in the evolution of artificial intelligence. OpenAI’s ambitious venture into developing an AI system with human-like reasoning capabilities – an endeavor epitomized by Qualia – underscores the significant strides being made towards the realization of artificial general intelligence (AGI). However, as we’ve examined, this advancement is not without its complexities and controversies.
The ethical and safety considerations surrounding Qualia, especially in the context of its potential to surpass human cognitive abilities, pose critical questions for the future of AI development. The internal debates within OpenAI, coupled with the broader discussions in the AI community, reflect the diverse perspectives and concerns regarding the rapid progression of such powerful technologies.
Moreover, OpenAI’s transition from a non-profit organization to a more commercially-driven entity, with Project Q* at its helm, signals a significant shift in the landscape of AI development. This raises pivotal questions about balancing the pursuit of technological advancement with responsible innovation and ethical stewardship.
Looking ahead, Project Q* stands at the forefront of a new era in AI – one that promises extraordinary advancements but also demands rigorous scrutiny and thoughtful consideration of its broader implications. As we venture deeper into this uncharted territory, the journey of Qualia will undoubtedly continue to shape our understanding and approach towards AGI, posing as both a beacon of possibilities and a mirror reflecting our deepest ethical and existential concerns.
In conclusion, Project Q* (Qualia) not only marks a milestone in AI’s capabilities but also serves as a catalyst for pivotal discussions about the future role of AI in society. Its development will likely continue to be a subject of intense study, debate, and fascination as we navigate the intricate interplay between technological innovation and ethical responsibility in the quest for AGI.