Credit Acceptance Corporation (CAC) is a publicly traded auto finance company focused on providing vehicle loans to customers with limited or impaired credit histories. Established in 1972, CAC’s model enables credit-challenged consumers to purchase vehicles, offering them a pathway to credit improvement by reporting timely loan payments to credit bureaus.
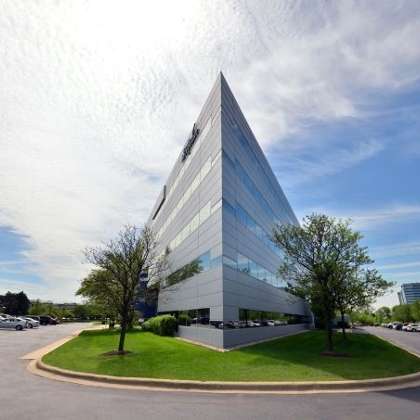
Mission and Market Position:
- Core Customer Base: CAC primarily serves subprime and credit-invisible consumers who often cannot secure loans through traditional financial institutions. This focus has allowed CAC to capture a unique market segment, providing financing options for individuals typically underserved in the auto finance sector.
- Dealer Partnerships and Financing Programs: CAC operates through two main programs:
- Portfolio Program: CAC advances funds to dealers and allows them to share in the loan’s cash flow, which incentivizes dealers to sell reliable vehicles that align with the customer’s ability to pay.
- Purchase Program: CAC buys loans outright from dealers, assuming responsibility for servicing and collection. These programs create revenue opportunities for dealers and broaden consumer access to vehicle ownership, while also sharing some risk with participating dealers(2023 Annual Report)(About Credit Acceptance).
Integration of Technology and AI/ML:
- Data Utilization in Operations: CAC relies heavily on data analytics to inform its loan underwriting, risk assessment, and customer management processes. The company gathers extensive data on credit behaviors and vehicle loan performance, which is analyzed to refine its predictive models. This data-centric approach has made CAC a strong player in an industry where data accuracy and predictive insights are critical to managing risk(2024-Oct-31-CACC.OQ-139…)(Credit Acceptance Annou…).
- AI/ML in Loan Underwriting: AI and ML tools support CAC’s underwriting processes by enhancing loan evaluation and risk management. Machine learning models assess customer risk profiles and help CAC structure loan terms to balance risk while extending financing opportunities to underserved customers. These AI-driven methods also support CAC’s ability to adjust for changes in credit trends or economic conditions(Job Posting – Leader of…)(Job Posting – Principal…).
- Application in Customer and Dealer Interactions: CAC applies AI and ML to improve both customer and dealer experiences. Automated tools assist in customer onboarding, provide streamlined access to loan information, and support the loan servicing process. For dealers, AI-driven interfaces simplify financing options, making the process more efficient and enabling real-time tracking of loan performance and customer status(Credit Acceptance Open …).
Strategic Positioning:
- Growing Demand in Subprime Auto Finance: The U.S. auto finance market, particularly in the subprime sector, is expanding due to recent shifts in credit availability. CAC’s data-driven and AI-enhanced approach positions it to capture this growing demand, especially as traditional lenders continue to impose stricter credit standards. CAC’s AI tools enable better borrower assessment, giving it a competitive edge in meeting the needs of subprime borrowers while managing financial risk(2023 Annual Report)(2024-Oct-31-CACC.OQ-139…).
- Focus on Responsible AI Practices: In response to regulatory and ethical considerations, CAC incorporates responsible AI practices into its technology strategy. By continuously refining its models for fairness and compliance, the company aims to avoid bias in lending decisions, supporting its long-term objective of ethical finance and responsible AI use(Job Posting – Leader of…)(Job Posting – Principal…).
Summary
CAC’s established presence in the subprime auto finance market is strengthened by its strategic focus on technology. AI/ML-driven underwriting and data analytics help optimize operations, mitigate risk, and improve customer and dealer services. As one of the leading companies in its sector, CAC’s approach combines established financing programs with advanced technology, positioning it to remain resilient in a complex market.
Financial Overview
Credit Acceptance’s financial performance is marked by steady growth in its loan portfolio, profitability through disciplined underwriting, and a strategic focus on long-term shareholder value via Economic Profit. This section highlights CAC’s recent financial results, capital strategy, and cost structure, supported by data from Q3 2024 earnings and recent annual reports.
Key Financial Metrics
Below is a summary of CAC’s financial performance for the third quarter and nine-month periods ending September 30, 2024:
Metric |
Q3 2024 |
Q2 2024 |
Q3 2023 |
9M 2024 |
9M 2023 |
GAAP Net Income ($ millions) |
$78.8 |
$(47.1) |
$70.8 |
$96.0 |
$192.5 |
GAAP Earnings per Share (Diluted) |
$6.35 |
$(3.83) |
$5.43 |
$7.68 |
$14.73 |
Adjusted Net Income ($ millions) |
$109.1 |
$126.4 |
$139.5 |
$352.9 |
$406.5 |
Adjusted Earnings per Share |
$8.79 |
$10.29 |
$10.70 |
$28.25 |
$31.10 |
Loan Portfolio (Adjusted, $ billions) |
$8.9 |
$8.6 |
$7.5 |
N/A |
N/A |
Initial Spread on Consumer Loans |
21.9% |
N/A |
21.4% |
N/A |
N/A |
Average Cost of Debt |
7.3% |
N/A |
5.8% |
N/A |
N/A |
Source: (Credit Acceptance Annou…)
Loan Portfolio and Collections Performance
CAC’s loan portfolio has consistently grown, reaching an adjusted $8.9 billion by Q3 2024—an 18.6% increase over the same quarter in 2023. Despite economic pressures, the company has maintained a strong collection performance, with adjustments reflecting changes in forecasted cash flows due to delinquency rates and macroeconomic conditions.
Loan Portfolio Growth |
Q3 2024 |
Q3 2023 |
Growth (%) |
Adjusted Loan Portfolio ($ billions) |
$8.9 |
$7.5 |
18.6% |
Loan Unit Growth |
+17.7% |
N/A |
N/A |
Dollar Volume Growth |
+12.2% |
N/A |
N/A |
CAC’s predictive models and conservative loan origination standards play a key role in managing loan performance. Despite these efforts, the 2021 and 2022 consumer loan vintages underperformed expectations, due largely to economic volatility and consumer spending pressures. The company recorded a modest 0.6% decline in forecasted net cash flows in Q3 2024, reflecting a $62.8 million decrease due to a higher-than-average decline in collection rates among certain loan segments(2024-Oct-31-CACC.OQ-139…)(Credit Acceptance Annou…).
Debt and Capital Management
CAC has adapted to the current interest rate environment by securing longer-term debt financing and expanding its revolving credit facilities. While the company’s average cost of debt increased to 7.3% in Q3 2024, CAC’s prudent capital management supports financial stability and future growth. Notably, the company has prioritized Economic Profit as a framework for evaluating capital allocation, focusing on shareholder value and sustainable profitability.
Debt Structure |
Q3 2024 |
Q3 2023 |
Average Cost of Debt |
7.3% |
5.8% |
Total Revolving Credit Facilities |
$1.6 billion |
N/A |
Unused Credit Capacity |
$1.4 billion |
N/A |
CAC’s recent debt restructuring included issuing higher-cost, long-term debt and maintaining a significant portion of available credit, which allows flexibility in funding while minimizing liquidity risks during periods of economic uncertainty. Additionally, CAC engaged in share repurchases, reducing common shares outstanding by approximately 4.5% since Q3 2023, indicating confidence in its long-term growth potential and commitment to Economic Profit(Credit Acceptance Annou…).
Forecasted Collection Rates
The following table highlights changes in forecasted collection rates across loan vintages. The collection forecast accuracy improves as loans age, providing CAC with insights into longer-term loan performance trends:
Consumer Loan Assignment Year |
Initial Forecast (%) |
Q3 2024 Forecast (%) |
Variance from Initial Forecast |
2015 |
67.7% |
65.3% |
-2.4% |
2016 |
65.4% |
63.9% |
-1.5% |
2017 |
64.0% |
64.7% |
+0.7% |
2021 |
66.3% |
63.8% |
-2.5% |
2022 |
67.5% |
60.6% |
-6.9% |
2023 |
67.5% |
64.3% |
-3.2% |
Source: (Credit Acceptance Annou…)
The 2021 and 2022 vintages have underperformed relative to initial forecasts, attributed primarily to post-pandemic economic shifts, supply chain issues, and higher inflation affecting consumer repayment capacity. These factors have influenced CAC’s focus on conservative underwriting and forecasting adjustments to maintain collection performance.
Profitability and Shareholder Returns
CAC continues to prioritize shareholder returns through Economic Profit, a measure that accounts for both the return on capital and cost of equity. Despite the volatility in recent loan vintages, CAC has generated substantial profitability, reflected in both GAAP and adjusted net income metrics. Stock repurchase efforts also align with this focus on maximizing Economic Profit per share.
In summary, CAC’s financial strategy emphasizes prudent growth, disciplined debt management, and AI-driven risk assessments, which together create a resilient financial foundation. By applying a long-term lens to its financial decisions, CAC remains well-positioned to navigate changing economic cycles while expanding its subprime auto finance portfolio.
Organizational Structure and Leadership
Credit Acceptance Corporation (CAC) maintains a structured organizational framework designed to support its mission of providing auto financing solutions to credit-challenged consumers. The company’s leadership team comprises seasoned professionals with extensive experience in finance, technology, and operations, ensuring effective governance and strategic direction.
Executive Leadership Team:
Position |
Executive |
Responsibilities |
Date Joined / Assumed Role |
Chief Executive Officer (CEO) and President |
Kenneth S. Booth |
Overall leadership and strategic direction of the company. |
Joined 2004, CEO since May 2021 |
Chief Financial Officer (CFO) |
Jay D. Martin |
Oversees financial operations, including accounting, financial reporting, and investor relations. |
Joined 2003, CFO since Jan 2024 |
Chief Operating Officer (COO) |
Jonathan L. Lum |
Manages day-to-day operations, ensuring efficiency and alignment with strategic goals. |
Joined 2002, COO since May 2019 |
Chief Technology Officer (CTO) |
Ravi Mohan |
Leads technology strategy, overseeing development and implementation of technological solutions. |
Joined Oct 2022 |
Chief Marketing and Product Officer |
Andrew K. Rostami |
Responsible for marketing strategies and product development. |
Joined Apr 2022 |
Chief People Officer |
Wendy A. Rummler |
Oversees human resources, focusing on talent acquisition, development, and maintaining company culture. |
Joined 2001, CPO since Sep 2022 |
Chief Legal Officer |
Erin J. Kerber |
Manages legal affairs and ensures compliance with laws and regulations. |
Joined 2010, CLO since Jul 2021 |
Chief Sales Officer |
Daniel A. Ulatowski |
Leads the sales department, focusing on dealer relationships and sales strategies. |
Joined 1996, CSO since Jan 2014 |
Chief Analytics Officer |
Arthur L. Smith |
Oversees data analytics to provide insights for business decision-making. |
Joined 1997, CAO since Aug 2013 |
Chief Treasury Officer |
Douglas W. Busk |
Manages treasury functions, including capital management and financial planning. |
Joined 1996, CTO since Jul 2020 |
Chief Alignment Officer |
Nicholas J. Elliott |
Ensures that company strategies and operations align with its goals. |
Joined 2005, CAO since Aug 2023 |
This structure supports Credit Acceptance Corporation’s mission to provide financing programs that enable auto dealers to sell vehicles to consumers, regardless of credit history.
Board of Directors:
CAC’s Board of Directors comprises individuals with diverse backgrounds in finance, law, and business management, providing oversight and strategic guidance to the company’s executive team. The board’s composition reflects a commitment to governance practices that align with shareholder interests and regulatory compliance.
Organizational Structure:
CAC’s organizational structure is designed to support its core business functions, including finance, operations, technology, legal, and compliance. The leadership team collaborates to implement the company’s strategic objectives, focusing on sustainable growth and customer satisfaction. This structure facilitates effective decision-making and operational efficiency, enabling CAC to adapt to market changes and maintain its position in the auto finance industry.
The company’s emphasis on technology, particularly in AI and ML, is evident in the leadership roles dedicated to these areas. The Chief Technology Officer oversees technological advancements, while the Chief Alignment Officer ensures that these initiatives align with the company’s strategic goals. This integrated approach allows CAC to leverage technology to enhance its services and operational capabilities.
Overall, CAC’s organizational structure and leadership are integral to its ability to provide financing solutions to credit-challenged consumers, maintain strong dealer relationships, and achieve financial performance objectives.
Credit Acceptance’s AI/ML Strategy and Technical Infrastructure
Credit Acceptance is leveraging advanced artificial intelligence (AI) and machine learning (ML) technologies to revolutionize its auto lending services. The company’s strategy involves building robust tools and services that enhance operational efficiency, improve customer experiences, and drive innovation. This overview explores the technical details of the tools and services being developed by the AI/ML teams, their applications, and the technologies underpinning them.
AI/ML Platforms and Services
End-to-End ML/AI Platforms
At the core of Credit Acceptance’s AI/ML infrastructure is a comprehensive platform designed to support the entire machine learning lifecycle. This platform provides services for:
- Data Ingestion and Processing: Tools that automate the collection, cleaning, and preprocessing of large datasets from various sources, ensuring high-quality data for model training (inferred based on standard practices for ML platforms).
- Feature Engineering and Feature Stores: Development of a centralized feature store that enables teams to create, store, and reuse features across different models, promoting consistency and reducing redundancy (explicitly mentioned in the job description as part of the ML/AI Platform team’s responsibilities).
- Model Development and Experimentation: Frameworks that allow data scientists and ML engineers to build, train, and experiment with models efficiently, including capabilities for hyperparameter tuning and version control of models (inferred as essential components of an ML platform).
- Model Deployment and Serving: Systems that facilitate the seamless deployment of models into production environments, supporting both batch and real-time inference, and ensuring scalability and reliability (inferred based on the mention of deployment and containerization in the job description).
- Monitoring and Maintenance: Tools that continuously monitor model performance, detect anomalies, and trigger alerts for data drift or degradation, enabling proactive maintenance and updates (inferred from the emphasis on monitoring in the job description).
Auto-Labeling Services
To accelerate the development of supervised learning models, the teams are building auto-labeling services that:
- Automate Annotation: Utilize pre-trained models and heuristics to label data automatically, reducing the manual effort required for data annotation (inferred from the mention of auto-labeling in the job description).
- Active Learning Integration: Implement active learning strategies where models identify and request labels for the most informative data points, improving model performance with less labeled data (inferred based on common practices in auto-labeling systems).
- Quality Assurance: Include validation processes to ensure the accuracy of auto-labeled data, leveraging human-in-the-loop systems for verification when necessary (inferred as a standard practice to maintain data quality).
Generative AI Workflows
The platform supports generative AI applications, focusing on:
- Large Language Models (LLMs): Development and fine-tuning of LLMs to support tasks such as natural language understanding, document processing, and conversational interfaces (inferred from the job description’s mention of experience with LLMs and Gen AI workflows).
- Custom Model Architectures: Designing models tailored to specific use cases within auto lending, such as generating personalized communications or summarizing customer interactions (inferred based on industry applications of generative AI).
- Integration with Business Processes: Embedding generative AI models into existing workflows, enhancing decision-making and automating complex tasks (inferred as a logical application of generative AI within the business).
Technical Details and Technologies
Cloud Infrastructure
Platform |
Services and Use Cases |
Source/Inferred |
Amazon Web Services (AWS) |
EC2 for compute resources, S3 for storage, and SageMaker for managed machine learning workflows. |
Inferred (based on AWS experience) |
Microsoft Azure |
Equivalent services to AWS for optimizing performance and cost. |
Explicitly mentioned as acceptable |
Google Cloud Platform (GCP) |
Equivalent services to AWS for optimizing performance and cost. |
Explicitly mentioned as acceptable |
Containerization and Orchestration
Tool |
Purpose |
Source/Inferred |
Docker |
Containerizes applications and services to create consistent environments across development, testing, and production. |
Inferred (standard with Kubernetes) |
Kubernetes |
Manages and orchestrates containers, handling scaling, load balancing, and deployment automation. |
Explicitly mentioned |
Programming Languages and Frameworks
Language/Framework |
Purpose |
Source/Inferred |
Python |
Primary language for ML development due to its extensive libraries and ease of use. |
Explicitly mentioned |
Java |
Used for performance-critical components and integrating ML services with existing enterprise systems. |
Explicitly mentioned |
C++ |
Also used for performance-critical components and integration with existing systems. |
Explicitly mentioned |
Machine Learning Libraries and Frameworks
Library/Framework |
Purpose |
Source/Inferred |
TensorFlow |
Used for developing deep learning models, particularly for neural networks and LLMs. |
Inferred (based on LLM mention) |
PyTorch |
Also used for deep learning models involving neural networks and LLMs. |
Inferred (based on LLM mention) |
Scikit-learn |
Applied for traditional ML algorithms and rapid prototyping/testing. |
Inferred (common in ML workflows) |
XGBoost |
Used for gradient boosting algorithms, effective for structured data in financial applications. |
Inferred (industry standard) |
LightGBM |
Another gradient boosting tool for structured data common in financial applications. |
Inferred (industry standard) |
Data Processing and Storage
Tool/Technology |
Purpose |
Source/Inferred |
Apache Spark |
Large-scale data processing, enabling distributed computing for big data. |
Inferred (common for big data) |
Hadoop Distributed File System |
Scalable storage solution for large datasets, commonly used alongside Spark. |
Inferred (common with Spark) |
SQL Databases |
Handle structured transactional data. |
Explicitly mentioned |
NoSQL Databases |
Manage unstructured data, such as logs and document storage. |
Explicitly mentioned |
DevOps and MLOps Tools
Category |
Tools/Technology |
Purpose |
Source/Inferred |
Version Control |
Git |
Ensures codebase integrity and supports collaboration. |
Inferred (standard practice) |
Continuous Integration |
Jenkins or GitLab CI/CD |
Automates testing, integration, and deployment pipelines for rapid releases. |
Inferred (emphasis on CI/CD) |
Experiment Tracking |
MLflow |
Manages the lifecycle of ML experiments, tracking parameters, metrics, and artifacts. |
Inferred (necessary for experiments) |
Model Serving and Deployment |
TensorFlow Serving or KFServing |
Provides high-performance serving of ML models, supporting RESTful APIs. |
Inferred (common for model serving) |
Monitoring and Logging |
Prometheus and Grafana |
Monitors system and application metrics, providing real-time insights. |
Inferred (standard monitoring practice) |
|
ELK Stack (Elasticsearch, Logstash, Kibana) |
Collects, indexes, and visualizes logs from applications and infrastructure. |
Inferred (widely used for logging) |
Applications and Use Cases
Risk Assessment and Credit Scoring
- Predictive Models: Develop sophisticated models that predict borrower risk, utilizing a variety of data sources, including credit history, employment data, and transactional behaviors (inferred based on typical applications in auto lending).
- Real-Time Decision Making: Implement models that provide instantaneous credit decisions, enhancing customer experience and operational efficiency (inferred as a logical application of ML in lending).
Customer Segmentation and Personalization
- Segmentation Algorithms: Use clustering and classification techniques to segment customers based on behaviors and preferences (inferred from common marketing strategies in the industry).
- Personalized Marketing: Leverage ML to tailor marketing campaigns and offers to individual customer profiles, increasing engagement and conversion rates (inferred as a practical application of AI/ML).
Fraud Detection and Prevention
- Anomaly Detection: Deploy models that identify unusual patterns indicative of fraudulent activities, using unsupervised learning techniques (inferred as standard practice in financial services).
- Real-Time Alerts: Integrate ML models with monitoring systems to trigger immediate alerts for suspicious transactions, enabling swift response (inferred based on industry practices).
Process Automation and Efficiency
- Document Processing: Use natural language processing (NLP) and computer vision to automate the extraction of information from documents like loan applications and identification forms (inferred as likely applications of AI in operations).
- Chatbots and Virtual Assistants: Implement AI-driven conversational agents to handle customer inquiries, providing instant support and reducing call center load (inferred from the mention of Generative AI workflows in the job description).
Predictive Maintenance and Operations
- System Health Monitoring: Apply ML to predict potential system failures or performance issues in IT infrastructure, enabling proactive maintenance (inferred as a use case for ML in IT operations).
- Resource Optimization: Use algorithms to optimize the allocation of computational resources, balancing cost and performance (inferred as a logical application to manage cloud resources effectively).
Team Structure and Collaboration
Role |
Responsibilities |
Source/Inferred |
Principal Engineers |
Lead technical design and architecture of ML platforms, mentor team members, and drive engineering excellence. |
Explicitly described in the job description |
Machine Learning Engineers |
Focus on model development, optimization, and deployment, ensuring models are production-ready. |
Inferred (based on standard team roles) |
Data Engineers |
Build and maintain data pipelines, ensuring data is accessible, reliable, and in the appropriate format for ML tasks. |
Inferred (essential for handling data) |
DevOps Engineers |
Manage CI/CD pipelines, automate deployments, and ensure infrastructure reliability. |
Inferred (mention of managing DevOps tools) |
Product Managers |
Define product roadmaps, gather requirements, and coordinate between technical teams and business stakeholders. |
Explicitly mentioned in Leader of ML/AI role |
Business Analysts and Domain Experts |
Provide industry insights, define business problems, and validate AI/ML solutions against business needs. |
Inferred (typical roles for business alignment) |
Collaboration Practices
- Agile Methodologies: Adopt Scrum or Kanban frameworks to manage work, with regular stand-ups, sprint planning, and retrospectives (preferred experience with Scrum and agile methodologies is mentioned in the job description).
- Cross-Functional Teams: Assemble teams with diverse skill sets to tackle specific projects, fostering innovation and holistic solutions (inferred from the “One Team” mindset emphasized in the competencies).
- Knowledge Sharing: Conduct regular workshops, code reviews, and brown-bag sessions to disseminate knowledge and best practices (inferred as standard practices to promote collaboration and learning).
Development Practices and Standards
Software Development Lifecycle (SDLC)
- Requirement Analysis: Collaborate with stakeholders to understand and document requirements (inferred from the emphasis on engaging with stakeholders in the job description).
- Design and Architecture: Create detailed design documents and architectural diagrams to guide development (inferred as part of driving technical strategy).
- Implementation: Follow coding standards, write clean and maintainable code, and conduct unit testing (explicitly required in the job description with an emphasis on production-quality code and code quality standards).
- Testing and Quality Assurance:
- Automated Testing: Implement unit, integration, and end-to-end tests to ensure functionality and performance (inferred as part of best practices in SDLC).
- Code Reviews: Perform peer reviews to maintain code quality and share knowledge (inferred as a common practice in engineering teams).
- Deployment: Utilize CI/CD pipelines to deploy applications and models in a controlled and repeatable manner (explicitly mentioned in the job description’s requirements for understanding CI/CD).
- Maintenance and Monitoring: Continuously monitor applications, address bugs, and update models as necessary (inferred from the focus on operational excellence and monitoring).
Security and Compliance
- Data Privacy: Implement strict access controls, data anonymization, and encryption to protect sensitive information (inferred from the need to simplify privacy compliance and responsible AI principles mentioned in the job description).
- Regulatory Compliance: Ensure all AI/ML solutions comply with industry regulations such as GDPR or CCPA, especially regarding data handling and customer rights (inferred as essential for any financial services company).
- Responsible AI Practices: Incorporate fairness, transparency, and explainability into models, reducing biases and building trust (explicitly mentioned as part of designing an AI platform adhering to responsible AI principles).
Innovation and Continuous Improvement
Staying Ahead with SOTA Technologies
- Research and Development: Allocate resources for exploring new algorithms, technologies, and methodologies (inferred from the job description’s emphasis on following industry and academic developments).
- Partnerships and Collaboration: Engage with academic institutions, industry groups, and technology vendors to stay informed of advancements (inferred as common practice for organizations aiming to adopt cutting-edge technologies).
- Internal Innovation Programs: Encourage team members to propose and work on innovative ideas that could benefit the company (inferred as part of fostering an innovative culture).
Professional Development
- Training and Workshops: Provide access to online courses, certifications, and conferences (inferred as part of the company’s focus on professional development and continuous improvement).
- Mentorship Programs: Pair junior team members with experienced professionals to foster growth (explicitly mentioned in the job description as mentoring junior engineers and interns).
- Knowledge Sharing Platforms: Utilize internal wikis, documentation, and forums for collaborative learning (inferred as standard practice to facilitate knowledge sharing).
Impact on Business and Customers
Enhanced Decision-Making
- Data-Driven Insights: Utilize AI/ML to uncover patterns and trends that inform strategic decisions (inferred as a fundamental benefit of AI/ML implementation).
- Risk Mitigation: Improve risk assessment models to reduce defaults and optimize lending portfolios (inferred based on applications in risk assessment and credit scoring).
Improved Customer Experience
- Personalization: Offer tailored products and services, enhancing customer satisfaction and loyalty (inferred from the potential applications in customer segmentation and personalization).
- Faster Services: Streamline processes like loan approvals and customer support, reducing wait times (inferred as a result of process automation and efficiency improvements).
Operational Efficiency
- Cost Reduction: Automate routine tasks, freeing up resources and reducing operational costs (inferred as a benefit of AI/ML automation emphasized in the job description’s focus on cost management).
- Scalability: Build systems that can handle increasing volumes without a proportional increase in costs (explicitly mentioned as a goal to design for scalability and cost efficiency).
Conclusion
Credit Acceptance’s commitment to integrating AI/ML technologies is transforming its operations and positioning it as an innovator in the auto lending industry. By building sophisticated tools and services, the company enhances its ability to make informed decisions, improve customer experiences, and operate efficiently. The deep technical expertise of its teams, combined with a culture of collaboration and continuous improvement, ensures that Credit Acceptance remains at the forefront of technological advancements, delivering significant value to both the business and its customers.
Customer Satisfaction Analysis and Improvement Analysis
Overview of Customer Feedback and Key Pain Points
Credit Acceptance Corporation’s customer feedback analysis, based on 1,490 Google reviews, provides insight into patterns of dissatisfaction that point to possible issues in the company’s approach to loan servicing and customer engagement. A significant proportion of the feedback highlights customer frustrations around four main areas: financial terms (interest rates and fees), transparency, customer service, and billing and payment processes. While some customers appreciate the company’s willingness to offer loans to individuals with limited or poor credit histories, these positive comments are few and often offset by the difficulties experienced during loan repayment.
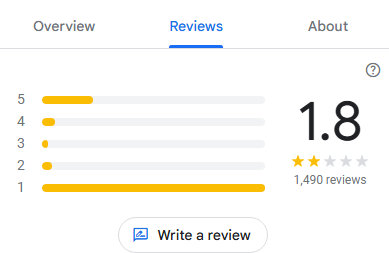
Many customers report that the company’s loan products, while accessible, come with high costs and risks that may not be initially clear. This dynamic, in turn, creates a feeling of entrapment, as customers find themselves facing mounting financial strain with limited support or relief options. The following analysis delves deeper into the primary pain points based on the reviews, with a focus on specific customer grievances and potential causes.
Key Pain Points
High Interest Rates and Fees
Customer Experience: High interest rates are one of the most frequently cited complaints, with some customers noting interest rates upwards of 20% or more. Many customers report paying far more than the original value of the vehicle over the course of their loan, which they see as disproportionate and exploitative. Additionally, fees related to early repayment or penalties for missed payments add to the financial burden, leaving customers feeling trapped in loans that are difficult to pay off.
Underlying Issues:
-
- Interest Rate Setting: The elevated interest rates reflect the higher credit risk of the customer base that Credit Acceptance serves. However, customers are often not prepared for how significantly these rates will impact their monthly payments and overall cost.
- Transparency in Loan Terms: Some customers report feeling misled about the total cost of the loan or the structure of interest payments. This lack of clarity in loan terms may point to a need for improved transparency and communication about the financial implications of high-interest loans.
- Fee Structure: In addition to high-interest rates, various fees related to payment processing, late payments, or early payoff create a compounding effect, increasing the overall debt burden. Customers often express frustration with these fees, as they exacerbate the financial strain and make loan repayment challenging.
Billing and Payment Issues
Customer Experience: Many customers cite problems with billing accuracy and payment processing, such as unauthorized withdrawals, unclear billing statements, and fees that appear unexpectedly. A particularly common issue is the difficulty in setting up and managing auto-pay services, which leads to unexpected withdrawals and disruptions in budgeting for customers. Additionally, some customers report that even when they attempt to make early payments, they are hit with penalties or experience issues with having the payments accurately reflected in their account statements.
Underlying Issues:
-
- Inconsistent Billing and Payment Systems: Issues with billing accuracy may stem from outdated or poorly integrated payment systems, leading to billing discrepancies, unexpected fees, and a lack of control for customers over their payment schedules.
- Unauthorized Withdrawals: Customers report unauthorized withdrawals or incorrect debits, which can be the result of technical errors in payment processing systems or lack of thorough customer support during payment setups.
- Lack of Payment Flexibility: Given the high interest rates, customers are often in a financially precarious position, and unexpected fees or penalties for payment adjustments only compound their financial stress. There may be insufficient options for payment flexibility, such as the ability to adjust payment schedules or handle partial payments without penalties.
Poor Customer Service
Customer Experience: A large portion of negative feedback centers around the quality of customer service interactions, with many customers describing their experiences as frustrating, time-consuming, and ineffective. Customers report being bounced from one representative to another without resolution and describe service representatives as unresponsive, unhelpful, or even rude. Long hold times, unclear responses, and inconsistency in handling issues leave customers feeling unsupported and devalued.
Underlying Issues:
-
- Customer Service Training and Empowerment: It is possible that customer service representatives are not adequately trained to handle complex loan-related inquiries or empowered to resolve issues independently. The resulting delays and confusion lead to a poor customer experience.
- High Call Volume and Wait Times: The company may lack sufficient staffing or streamlined call management, leading to longer wait times and customers being passed between representatives.
- Lack of Escalation Paths: Customers often express frustration at not being able to reach someone who can make decisions or offer solutions. Without a clear escalation process, customers may feel stuck with representatives who lack authority to address their concerns, especially in cases of billing disputes or payment adjustments.
Deceptive Lending Practices
Customer Experience: Many customers allege that the loan terms were presented differently at the point of sale compared to what they eventually agreed to, or that dealership representatives were unclear or misleading in explaining the terms of their loan agreements. These complaints often focus on the lack of transparency regarding interest rates, fees, and the overall cost of the loan. Customers report feeling misled about the true financial implications of their agreements and are particularly frustrated by how the loan terms seem to shift or become less favorable post-signature.
Underlying Issues:
-
- Inconsistent Communication Across Sales Channels: Some of the issues may arise from differences in communication between Credit Acceptance and the dealerships that offer its financing. Dealership representatives might not accurately or consistently convey loan terms, leading to customer dissatisfaction when terms are clarified later.
- Complex Loan Terms: The loan terms, including fees and penalties, may be complex and not thoroughly explained at the time of signing, leaving customers to discover additional costs only when they receive their statements or bills. Simplifying and clarifying these terms, particularly for high-risk loans, could help alleviate some of the issues.
- Predatory Perception: Due to high interest rates and aggressive fee structures, customers may view Credit Acceptance as a predatory lender, a perception that can be damaging in the long term. Customers with few credit options might feel they are being exploited rather than supported.
Additional Observations
1. Loan Restructuring Difficulties: Customers facing financial hardship report difficulties in obtaining support for loan restructuring or payment deferrals. Without options for assistance, customers who might otherwise remain loyal or successfully pay down their loans end up in situations where they feel unsupported.
2. Negative Impact on Credit Scores: Late fees and penalties are often reported as disproportionately impacting customers’ credit scores, leading to a cycle of debt and poor credit. Customers feel that they are penalized heavily for minor errors or misunderstandings, which not only strains their finances but also affects their future creditworthiness.
3. Communication Gaps and Documentation Issues: Many customers cite issues with receiving incomplete or delayed documentation, particularly around loan payoff statements, lien releases, and transaction confirmations. These gaps in communication often exacerbate customer frustrations, as they feel left in the dark about their financial obligations.
Summary of Key Areas for Improvement
Addressing these pain points requires a multi-faceted approach that includes improving billing transparency, enhancing customer service responsiveness, and ensuring consistent and transparent communication of loan terms at the point of sale. Leveraging AI and ML solutions in billing systems, customer service, and feedback analysis could help Credit Acceptance not only manage but also proactively address many of these challenges. With the right changes, Credit Acceptance can reposition itself as a fair and supportive lender, focusing on customer empowerment rather than dependency on high-interest debt.
Role of AI/ML in Addressing Pain Points
Leveraging AI and Machine Learning (ML) technologies can significantly improve the customer experience, operational efficiency, and decision-making capabilities for companies like Credit Acceptance Corporation. Focusing on practical, actionable applications within existing AI/ML frameworks and tools can directly address many of the common pain points reported by customers, particularly in areas of billing transparency, customer service, loan affordability, and proactive communication. Here’s a detailed analysis of how AI and ML can help resolve specific customer challenges using available tools and realistic implementations:
1. Predictive Analytics for Customer Risk Assessment and Personalized Loan Offers
Problem Addressed: High-interest rates and a lack of personalized loan terms are primary sources of customer dissatisfaction. Many customers feel burdened by loan terms that are not tailored to their financial situation, which increases their default risk and negatively impacts their long-term financial health.
AI/ML Solution: Implementing predictive analytics and machine learning algorithms for customer risk assessment can help Credit Acceptance Corporation better understand individual customers’ financial profiles. By analyzing historical data, income patterns, credit scores, and payment behaviors, an ML model can predict the likelihood of default and personalize loan terms accordingly. This enables more favorable terms for lower-risk customers, potentially reducing interest rates for those who can demonstrate good repayment behavior.
Toolset and Approach:
- Scikit-Learn and XGBoost: These ML libraries can help create and fine-tune models that predict repayment likelihood, allowing for more personalized interest rates and payment schedules.
- TensorFlow for Deep Learning: Complex customer profiles could be modeled using neural networks to capture nuanced relationships between customer attributes and repayment behavior.
- Data Sources and Integration: Using existing customer financial history and demographic data, these models can be integrated into the loan origination process to ensure more personalized and equitable loan offerings, thus reducing complaints about high-interest rates.
2. Automated Billing and Payment Management via Natural Language Processing (NLP) and Chatbots
Problem Addressed: Customers frequently report issues with billing accuracy, unexpected fees, and payment management. The confusion often leads to delays in payments, financial strain, and dissatisfaction with customer service interactions.
AI/ML Solution: Implementing NLP-powered chatbots and virtual assistants can help automate billing inquiries, payment scheduling, and dispute resolution. An AI-driven billing assistant could clarify fees, remind customers about upcoming payments, and offer flexible payment options without requiring extensive human intervention.
Toolset and Approach:
- Dialogflow and Amazon Lex: These NLP tools can be used to build chatbots that handle billing inquiries, explain fees, and offer options to defer or reschedule payments. For example, a chatbot could provide real-time information on why a particular fee was charged, or help customers set up auto-pay or make partial payments to avoid penalties.
- Speech Recognition APIs: Using speech-to-text APIs can improve accessibility for customers calling into service centers, enabling voice-activated billing inquiries and service requests without waiting for a representative.
- Automated Workflow Integration: Integrating these NLP-powered assistants with back-end billing systems can help ensure billing accuracy, timely notifications, and streamlined payment processes. Customers could access billing information through the chatbot or receive payment reminders tailored to their preferences, reducing friction and improving transparency.
3. Sentiment Analysis for Customer Feedback Management and Service Improvement
Problem Addressed: Customer feedback highlights dissatisfaction with service quality, including long wait times, unhelpful responses, and lack of issue resolution. These factors contribute to a negative perception of the company and erode trust.
AI/ML Solution: Applying sentiment analysis to customer reviews, call transcripts, and feedback surveys can identify common pain points in real-time and inform customer service improvements. By continuously analyzing sentiment trends, the company can proactively address recurring issues, train customer service representatives on specific challenges, and adjust service processes based on customer needs.
Toolset and Approach:
- Natural Language Processing with NLTK and TextBlob: Sentiment analysis tools like NLTK and TextBlob can process text feedback from reviews, surveys, and chat logs, categorizing feedback as positive, negative, or neutral and detecting specific themes like billing, customer support, or product dissatisfaction.
- Amazon Comprehend or Google Cloud NLP: These cloud-based NLP services can process large volumes of unstructured feedback data, extract keywords, and identify common issues without requiring extensive in-house data science resources.
- Automated Feedback Loop: Sentiment analysis can generate reports for customer service managers, highlighting top issues and suggesting areas for improvement. By regularly updating this feedback loop, service teams can be trained to better respond to identified concerns and improve response accuracy and empathy.
4. Fraud Detection and Security Enhancement Using Anomaly Detection Models
Problem Addressed: Unauthorized withdrawals and billing discrepancies create financial stress for customers and contribute to distrust in the company’s payment handling. Addressing these issues requires robust security and fraud prevention measures to protect customer data and payment integrity.
AI/ML Solution: Anomaly detection models can flag unusual payment activity, such as unauthorized transactions or unexpected fees. These models work by identifying deviations from a customer’s normal transaction patterns, allowing for proactive investigation of potential billing errors or fraudulent activity.
Toolset and Approach:
- Isolation Forest and One-Class SVM: These ML algorithms are particularly effective for detecting anomalies in transaction data. By training on regular transaction histories, they can detect and flag suspicious activities for further investigation.
- AWS SageMaker or Azure Machine Learning: Using cloud-based ML platforms, the company can develop anomaly detection models at scale, integrating them directly with billing systems to continuously monitor transactions and flag irregularities.
- Real-Time Alerting System: Integrating anomaly detection with real-time alerting allows for immediate response to flagged transactions. Customers could be notified of potential issues before they escalate, and any unauthorized withdrawals or billing discrepancies can be resolved promptly.
5. Enhanced Loan Document Processing Using Optical Character Recognition (OCR) and Document Parsing
Problem Addressed: Many customers experience confusion regarding loan terms and report feeling misled about interest rates, penalties, and other loan conditions. Clear communication of loan terms and quick access to documentation are essential for customer satisfaction.
AI/ML Solution: OCR and document parsing technologies can digitize and analyze loan documents to ensure consistent and transparent communication of terms. This automation helps reduce errors, improve access to loan information, and ensure customers are fully informed of their loan obligations.
Toolset and Approach:
- Tesseract OCR: This open-source tool can digitize loan documents, making them searchable and analyzable for consistency across customer interactions.
- Document AI from Google Cloud or Amazon Textract: These tools offer advanced OCR and data extraction capabilities, enabling automated parsing of complex loan documents. By extracting key terms and conditions, the company can highlight crucial information like interest rates, fees, and penalties, making it easier for customers to understand their obligations.
- Automated Term Highlighting: Loan documents processed through OCR and document parsing can be integrated with customer service interfaces. Representatives and chatbots can access specific loan terms instantly, providing clear explanations to customers without delays, thereby reducing confusion and dissatisfaction.
6. Proactive Customer Engagement Through Personalized Communication
Problem Addressed: Lack of proactive engagement leaves customers feeling unsupported, especially when they encounter financial challenges or require adjustments to their loan terms. Timely, personalized communication can alleviate these frustrations.
AI/ML Solution: Using customer segmentation models, Credit Acceptance can send targeted, personalized messages to customers based on their payment history, risk profile, and current financial circumstances. By anticipating customer needs, the company can offer support options proactively, such as temporary payment deferrals, adjustments, or alternative payment plans.
Toolset and Approach:
- K-Means Clustering for Customer Segmentation: Clustering algorithms can group customers based on payment behaviors, financial stress indicators, and past interactions, allowing for more tailored communication strategies.
- Automated Campaigns Using ML-Driven Recommendation Systems: A recommendation system could suggest optimal engagement strategies, such as offering payment plan adjustments or sending reminders for customers who show signs of financial distress.
- Integration with CRM Platforms: AI-driven insights from customer segmentation can be integrated into CRM systems to support personalized outreach efforts. Representatives can access these insights to provide more relevant assistance, ensuring each customer receives individualized support and reducing the perception of neglect.
Conclusion
Credit Acceptance Corporation can leverage a suite of AI/ML tools and techniques to address its primary customer pain points in a cost-effective and practical manner. Predictive analytics, NLP-powered customer support, sentiment analysis, fraud detection, and document processing can all be realistically implemented to improve customer satisfaction and operational efficiency. By prioritizing these AI/ML solutions, the company can enhance transparency, reduce financial stress, and foster more supportive and meaningful relationships with its customers. This targeted use of technology can transform high-risk lending into a more customer-centered and financially stable experience, ultimately benefiting both the company and its clients.
References
Credit Acceptance Careers. “Principal Engineer – ML AI Platform and Leader of ML/AI Solutions.” Workday Jobs. Credit Acceptance Corporation, https://creditacceptance.wd5.myworkdayjobs.com/en-US/Credit_Acceptance/.
Credit Acceptance Corporation. About Credit Acceptance. Credit Acceptance, https://www.creditacceptance.com/about.
Credit Acceptance Corporation. 2023 Annual Report. Credit Acceptance, https://www.ir.creditacceptance.com/static-files/4b6ba102-3fef-4676-b82e-13eddb388d8c.
Credit Acceptance Corporation. “Q3 2024 Earnings Call.” 31 Oct. 2024, Credit Acceptance Corp Investor Relations, https://www.ir.creditacceptance.com/static-files/01c2a0cb-ccf7-4f6c-8fa4-830584e2f566.
Credit Acceptance Corporation. Third Quarter 2024 Results. Credit Acceptance, https://www.ir.creditacceptance.com/static-files/01c2a0cb-ccf7-4f6c-8fa4-830584e2f566.
Credit Acceptance Corporation. “3rd Quarter 2024 Earnings Call.” MediaServer, https://edge.media-server.com/mmc/p/j5xf32kd/.
Credit Acceptance Corporation. SEC Filings. U.S. Securities and Exchange Commission, https://www.sec.gov/Archives/edgar/data/885550/000088555024000119/0000885550-24-000119-index.htm.
Credit Acceptance Corporation. Press Releases. Credit Acceptance, https://www.ir.creditacceptance.com/press-releases.
Credit Acceptance Corporation. Google Reviews. Google, https://www.google.com/maps/place/Credit+Acceptance/.